An expert’s guide to image generation with DALL-E 3
With its impressive capabilities, DALL-E 3 is revolutionizing the way we think about image generation.
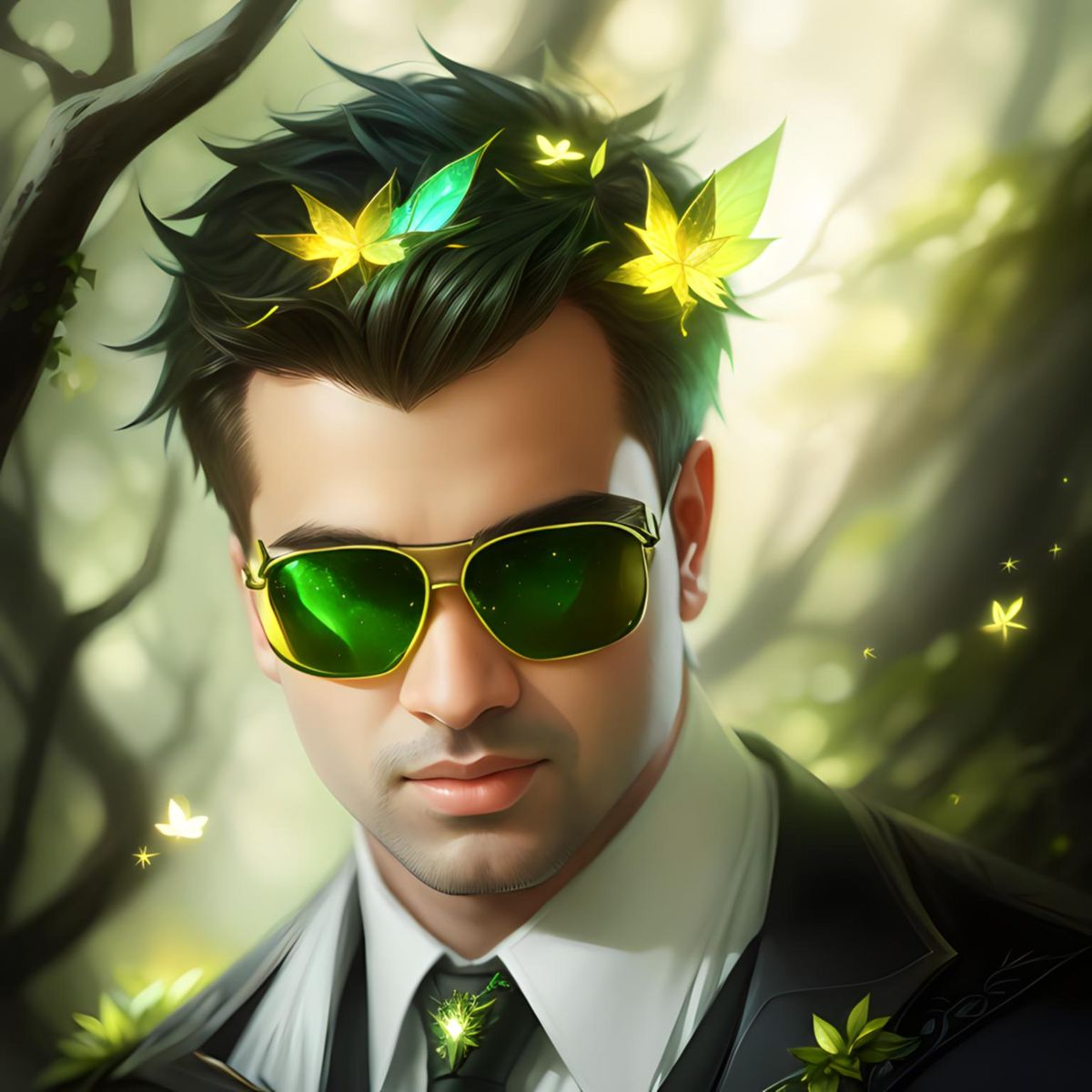
Introduction to DALL-E 3
DALL-E 3 is an advanced image generation model developed by OpenAI. It utilizes deep learning techniques to generate highly realistic and diverse images from textual descriptions. Unlike traditional image generation methods, DALL-E 3 can create images that have never been seen before.
This groundbreaking technology opens up a wide range of possibilities in various fields such as art, design, and advertising. With its impressive capabilities, DALL-E 3 is revolutionizing the way we think about image generation.
How DALL-E 3 works
DALL-E 3 is an advanced image generation model that utilizes deep learning techniques. It operates by combining a transformer-based encoder-decoder architecture with a discrete latent space. The model is trained on a large dataset of images and their corresponding text descriptions. During the generation process, DALL-E 3 uses the learned representations to map textual prompts to visual outputs. This enables it to generate highly realistic and novel images based on given textual inputs. The use of a discrete latent space allows for precise control over the generated images, enabling users to specify desired attributes and characteristics. The combination of these techniques makes DALL-E 3 a powerful tool for image generation.
Applications of DALL-E 3
DALL-E 3 has a wide range of applications in various fields. It can be used for creative design, artificial intelligence research, and content generation. With its ability to generate high-quality images based on textual descriptions, DALL-E 3 opens up new possibilities for visual storytelling, advertising, and virtual reality experiences. Its versatility and flexibility make it a valuable tool for graphic designers, game developers, and content creators. Moreover, DALL-E 3 can also be applied in data augmentation, image synthesis, and image editing tasks, providing a powerful solution for enhancing existing datasets and generating new visual content.
Image Generation Techniques
Traditional image generation methods
Traditional image generation methods involve techniques such as painting, drawing, and photography. These methods require manual effort and artistic skills to create visually appealing images. However, they have limitations in terms of scalability and flexibility. Additionally, traditional methods may not be able to generate images that go beyond what already exists in the real world. In contrast, DALL-E 3, a deep learning-based image generation model, offers a revolutionary approach to creating unique and imaginative images. It can generate images that are completely novel and have never been seen before, pushing the boundaries of visual creativity.
Deep learning-based image generation
Deep learning-based image generation techniques have revolutionized the field of computer vision. These methods leverage the power of neural networks to generate highly realistic and diverse images. One of the most groundbreaking advancements in this domain is DALL-E 3, a state-of-the-art image generation model developed by OpenAI. DALL-E 3 combines the power of deep learning with the ability to understand and generate images from textual descriptions. It uses a transformer-based architecture that allows it to capture complex patterns and generate high-quality images. Compared to traditional image generation methods, DALL-E 3 offers unparalleled flexibility and creativity. It can generate images that are not only visually appealing but also conceptually coherent.
This makes DALL-E 3 a powerful tool for various applications, including art, design, and visual storytelling.
Comparison of DALL-E 3 with other techniques
DALL-E 3 revolutionizes image generation by combining traditional methods with deep learning techniques. Unlike traditional methods that rely on predefined templates or handcrafted features, DALL-E 3 learns to generate images from scratch based on a massive dataset. This allows DALL-E 3 to create highly realistic and diverse images that were previously impossible to achieve. Compared to other deep learning-based image generation models, DALL-E 3 stands out with its ability to generate contextually relevant images and its unprecedented control over the generated output.
Here are the limitations of DALL-E:
- Contextually relevant image generation
- Unprecedented control over the output | - Requires a massive dataset for training
- Computationally intensive
- Limited interpretability | | Other techniques | - Simplicity in training
- Faster inference time
- Interpretable models | - Limited diversity in generated images
- Lack of control over the output
- Less realistic results
Training DALL-E 3
Data collection and preprocessing
Data collection and preprocessing are crucial steps in training DALL-E 3. To collect a diverse dataset, a large number of images from various sources are gathered. These images are then preprocessed to ensure uniformity in size, resolution, and format. Additionally, the dataset is carefully curated to remove any irrelevant or low-quality images. The preprocessing step also involves extracting relevant metadata, such as image captions or tags, which can be used during the training process. Overall, the quality and diversity of the dataset greatly influence the performance of DALL-E 3 in generating high-quality and diverse images.
Architecture of DALL-E 3
The architecture of DALL-E 3 is based on a transformer neural network, which allows it to generate high-quality images with fine details. The network consists of an encoder that encodes the input text into a latent space representation and a decoder that generates the corresponding image from the latent space. The transformer architecture enables DALL-E 3 to capture complex relationships between the text and image domains, resulting in impressive image generation capabilities. Additionally, DALL-E 3 utilizes conditional generation, allowing users to specify desired attributes or constraints for the generated images. This architecture, combined with the vast amount of training data, contributes to the remarkable performance of DALL-E 3 in image generation tasks.
Training process and optimization
The training process of DALL-E 3 involves several steps to optimize the model's performance. Firstly, a large dataset of images is collected and preprocessed to ensure high-quality input data. The architecture of DALL-E 3 is designed to handle the complexity of image generation tasks, with layers of neural networks and attention mechanisms. During training, the model learns to generate images by minimizing a loss function through optimization techniques such as stochastic gradient descent. The optimization process iteratively adjusts the model's parameters to improve its ability to generate realistic and diverse images. Through careful training and optimization, DALL-E 3 achieves impressive results in image generation, surpassing traditional methods and even other deep learning-based techniques.
Conclusion
Advantages and limitations of DALL-E 3
DALL-E 3 offers several advantages in image generation, including the ability to create highly detailed and realistic images from textual descriptions. It also allows for the generation of novel and unique images that have never been seen before. However, there are also some limitations to consider. DALL-E 3 requires a large amount of training data and computational resources, making it inaccessible for many users. Additionally, the generated images may not always align perfectly with the intended description, leading to some degree of ambiguity. Despite these limitations, DALL-E 3 represents a significant advancement in the field of image generation, with the potential for exciting applications in various domains.
Prospects of image generation with DALL-E 3
The prospects of image generation with DALL-E 3 are promising. As the technology continues to advance, we can expect improvements in the quality and diversity of generated images. DALL-E 3 has the potential to revolutionize various industries, including advertising, entertainment, and design. With its ability to create unique and imaginative visuals, it opens up new possibilities for artists, marketers, and content creators. However, there are also challenges to overcome, such as ethical considerations and the need for more efficient training methods. Overall, the future of image generation with DALL-E 3 holds great potential for innovation and creativity.
Closing thoughts
In conclusion, DALL-E 3 is a groundbreaking image generation model that combines traditional and deep learning-based techniques. Its ability to generate highly realistic and diverse images opens up a wide range of applications in various fields. However, it is important to note that DALL-E 3 also has its limitations, such as the need for extensive training and the potential for bias in the generated images.
Nonetheless, with further advancements and improvements, DALL-E 3 holds great promise for the future of image generation. Exciting times lie ahead!