AI Trends in Marketing - The Complete Evolution
This eBook looks at how AI is evolving marketing and shaking up the industry.
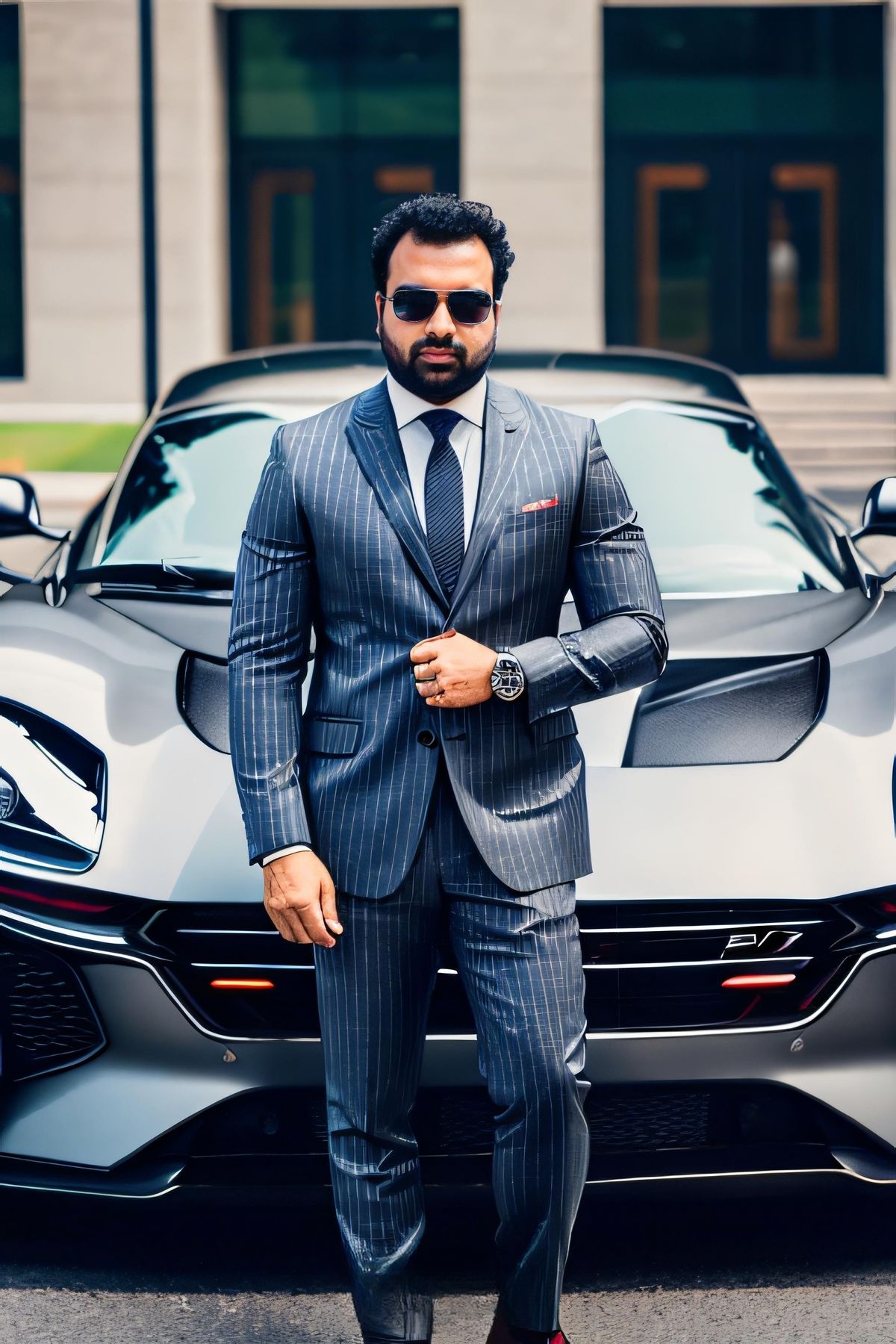
Artificial intelligence is changing marketing in major ways. As AI keeps advancing quickly, marketers are using this tech to be more efficient, personalized and innovative across marketing.
This eBook by the Human AI "Digital Marketing Legend - Srinidhi Ranganathan" looks at how AI is evolving marketing and shaking up the industry.
Table of Contents:
Chapter 1 defines artificial intelligence and machine learning, explaining how they're different and providing a short history of how these technologies have advanced over time. We look at early theoretical work in the 1950s and 1960s, the creation of neural networks and deep learning, and some big AI achievements in the 21st century. This background gives context for current AI uses in marketing.
Chapter 2 explores key AI technologies powering marketing change today - machine learning, natural language processing, computer vision, speech recognition, chatbots and virtual agents. For each, we explain definitions, and real examples of marketing applications, and discuss capabilities and limits. This tech foundation sets up the AI marketing trends in the rest of the eBook.
Chapter 3 examines how AI enhances marketing analytics and provides insights from customer data. Marketers can now track and analyze massive amounts of data to spot trends, predict customer behaviour, personalize content, and optimize campaigns in real time. AI makes data more actionable through machine learning, predictive analytics, sentiment analysis, and propensity modelling. However, AI raises data privacy concerns that marketers must consider.
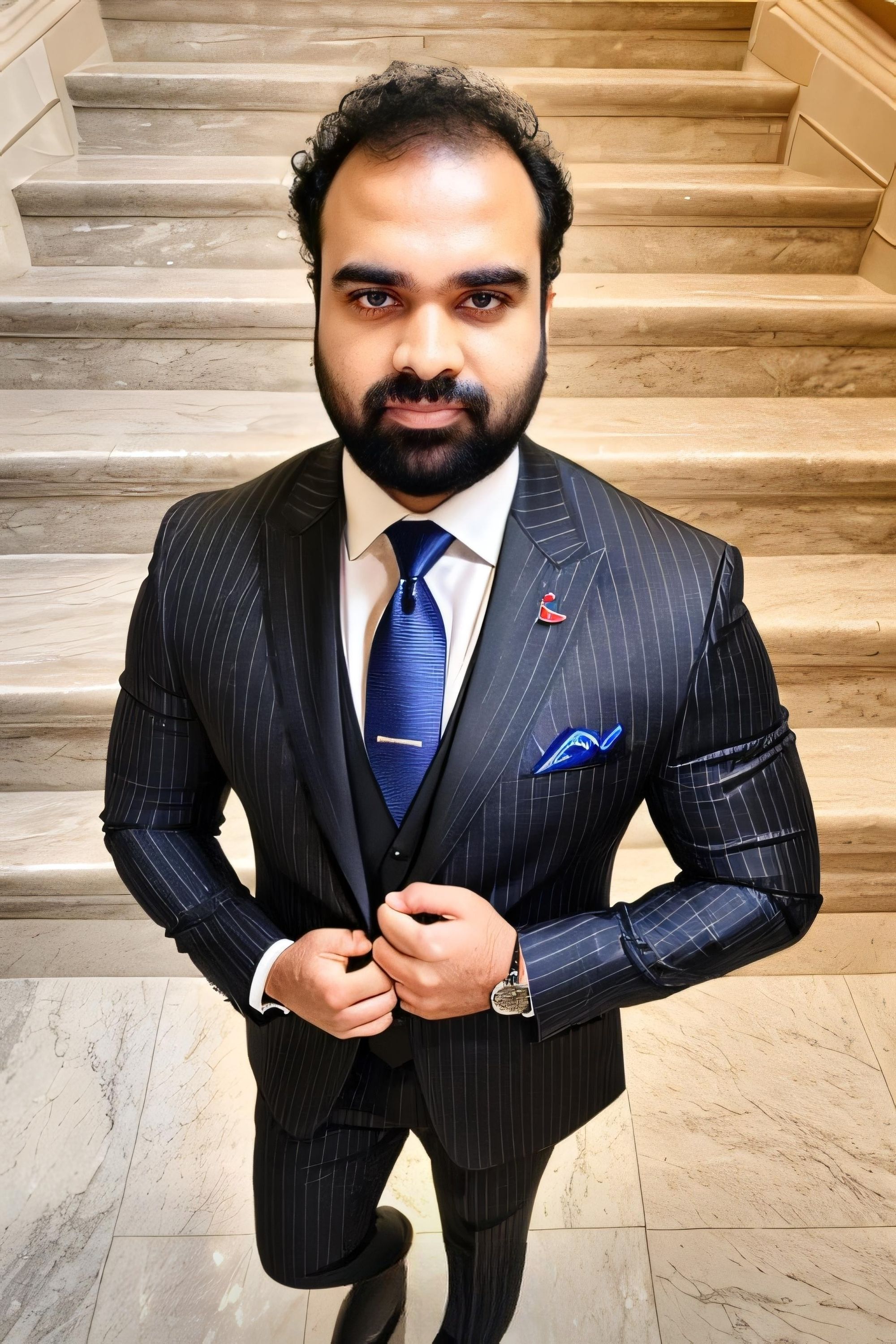
Moving from behind-the-scenes to customer-facing apps, Chapter 4 looks at how AI is changing content creation and delivery. Natural language generation systems customize content for specific audiences. Chatbots provide 24/7 personalized customer service. And dynamic creative optimization uses AI to iterate ad creative in real-time to improve campaign performance. However, marketers need to ensure AI-generated content aligns with brand voice.
Chapter 5 explores AI's role in search engine optimization (SEO) and marketing automation. AI can optimize websites for better search rankings by analyzing page content, keywords, links, and more. Marketing automation platforms use machine learning to score leads, segment audiences, and trigger personalized messaging. AI provides key insights to boost SEM and SEO results. But marketers should know Google's policies around auto-generated content.
Looking at sales applications, Chapter 6 explains how AI is transforming lead generation and conversion. AI can ID high-quality leads, provide sales recommendations, and autonomously nurture leads until sales-ready. However, using AI too aggressively may alienate customers. Marketers should ensure human accountability in AI systems.
Chapter 7 surveys emerging AI marketing applications like product recommendations, affective computing analyzing emotions, augmented reality, and voice search optimization. These new applications have huge potential.
Finally, Chapter 8 considers AI's broader implications for marketing. With human creativity augmented by machines, marketers can focus more on strategy, creativity and emotional connections. But marketers need new skills to maximize AI's potential, and ethical issues around bias, transparency and privacy must be addressed responsibly. Used properly, AI can take marketing to new heights.
In summary, this eBook looks at AI innovation's quickening pace in marketing. As machine learning evolves, AI is streamlining processes, providing insights, boosting personalization and enabling new apps. However, marketers must evaluate each AI technology to determine if, how, and when to implement it. This eBook aims to give a big-picture perspective on AI in marketing, helping readers understand current trends, real-world uses, key opportunities and challenges. AI is radically reshaping marketing, and savvy marketers who use these technologies effectively will gain a big competitive advantage. This transformation promises to be both disruptive and empowering for the field.
Chapter 1 - An Introduction to Artificial Intelligence and Machine Learning
Artificial intelligence (AI) refers to computer systems designed to perform tasks that normally require human intelligence. AI systems can perceive their environment, reason through problems, learn from experience and take action to achieve goals. AI research started in the 1950s, with cycles of hype and disappointment over the decades. But with major advances in data, computing power, algorithms, and storage, AI has made huge practical progress and investment recently.
Machine learning is one of the most important capabilities within AI. Machine learning algorithms can learn and improve at tasks through experience without explicit programming. They detect patterns in training data to make predictions or decisions. Instead of rules-based programming, machine learning develops models that get better iteratively.
This chapter will overview key concepts, history, and terminology for artificial intelligence and machine learning. This foundation will help readers understand the evolution and current landscape of AI in marketing covered later in the eBook.
Defining Artificial Intelligence
The term “artificial intelligence” was first used in 1956 at an academic conference. AI pioneer John McCarthy defined it as “the science and engineering of making intelligent machines.” Rather than manually coding intelligence into machines rule-by-rule, the goal of AI has been to develop algorithms and systems that can learn and reason like humans.
While a universally accepted definition is elusive, AI generally refers to systems that demonstrate human-like cognitive abilities including perceiving, reasoning, learning, problem-solving, and creativity. AI systems can process huge data volumes and identify patterns not apparent to humans. The algorithms continue improving through new data.
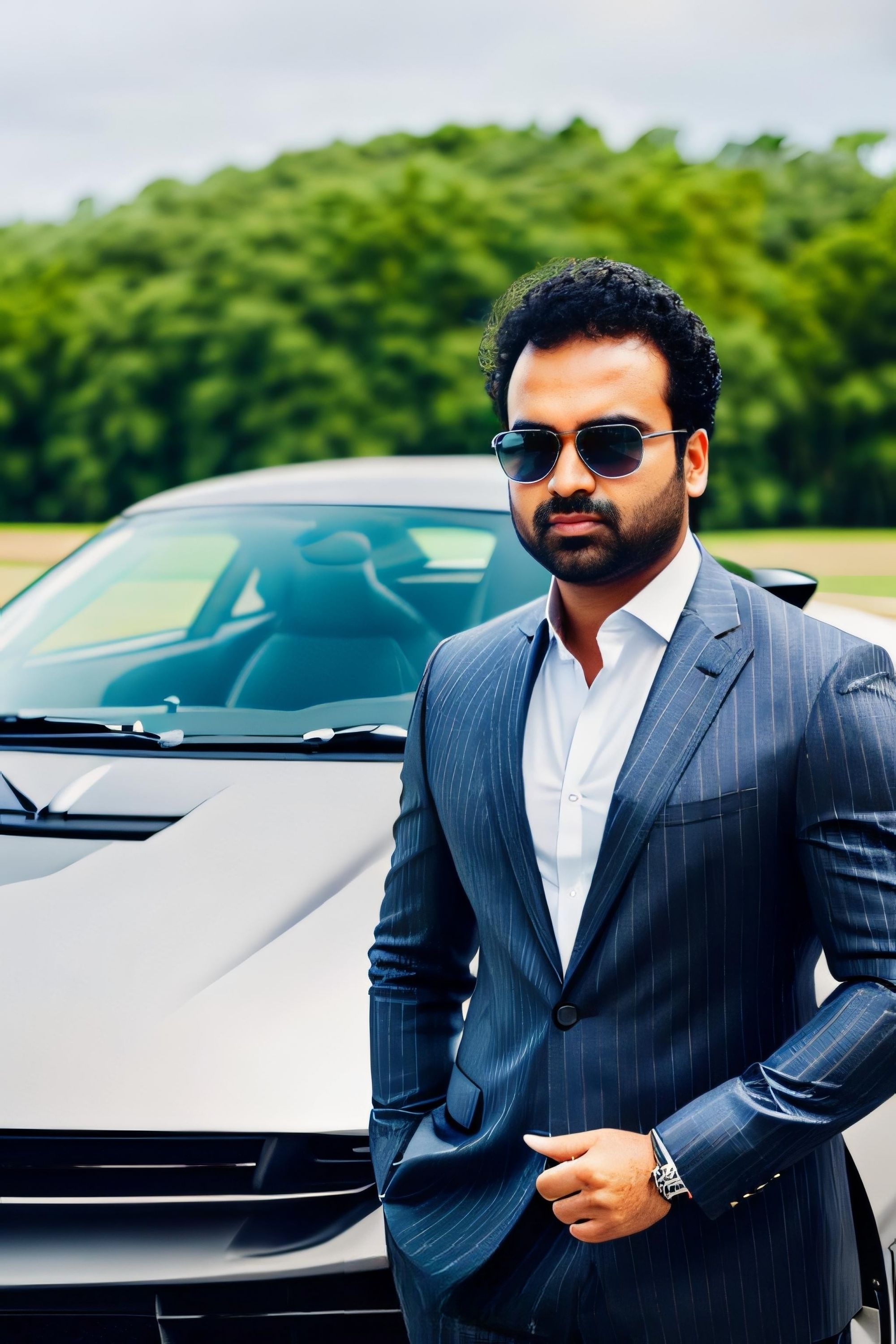
AI capabilities generally fall into two categories:
Narrow AI - Also called weak AI, involves systems designed for specific, narrowly defined tasks like virtual assistants, image recognition, fraud detection, and predictive maintenance. These systems perform focused functions extremely well but lack generalized intelligence.
Artificial General Intelligence (AGI) - Also known as strong AI, AGI aims to develop systems with generalized cognitive abilities at the human level and beyond. Achieving human-level AGI remains an ongoing scientific pursuit likely decades away.
Machine Learning Defined
As mentioned, machine learning focuses on algorithms that can learn without explicit programming. Instead of rules-based programming, machine learning relies on models trained on large example data sets to identify patterns and make predictions or decisions about new data.
There are several types of machine learning:
Supervised learning - Models are trained on labelled example data with the “right answers.” For instance, an image recognition model could be trained on cat and dog images. The model examines many images and learns the visual patterns that correlate with each label, then categorizes new images it hasn't seen.
Unsupervised learning - Models must derive insights from datasets without pre-labelled examples. The algorithm explores the structure and variable relationships within the data. Identifying customer segments from purchasing data is an example.
Reinforcement learning - Models learn through trial-and-error interactions with their environment, exploring actions to arrive at the optimal approach to maximize a reward. Game-playing and self-driving car navigation use reinforcement learning.
In practice, machine learning models often combine these approaches and various algorithms like decision trees and neural networks. We’ll explore some common algorithms later.
Brief History of AI
The pursuit of intelligent machines dates back centuries, with major strides in the 20th century. Here are some key AI milestones:
1943 - Warren McCulloch and Walter Pitts develop the first computational neural network model, establishing key AI concepts.
1950 - Alan Turing proposes the Turing Test to assess a machine's intelligence through natural language conversations.
1956 - The phrase “artificial intelligence” is coined at an academic conference launching AI as a field.
1959 - Arthur Samuel programs a checkers-playing computer, an early machine learning achievement. The IBM computer got better by analyzing games.
1961 - James Slagle develops an early AI program for solving geometric analogies.
1997 - IBM's Deep Blue beats world chess champion Garry Kasparov, showing rapid AI progress.
2011 - IBM's Watson defeats human champs on Jeopardy, demonstrating natural language understanding.
2012 - Deep learning breakthrough enables Google AI to recognize cats in YouTube videos with no human input.
2016 - Google DeepMind's AlphaGo beats the world champion in the complex game Go.
2020's - AI matches or exceeds human capabilities in more focused domains, enabling real-world applications. However, general human-level AI remains elusive.
AI has followed boom-bust cycles of hype and disappointment. Eventually, practical outcomes matured each AI winter. With today's data and computing power explosions, AI now appears advanced enough to drive progress across industries, including marketing.
Technical Foundations of AI
Now that we’ve defined key terms and traced AI's evolution, let’s look under the hood at how AI technologies actually work - neural networks, deep learning, and some common algorithms.
Neural Networks and Deep Learning
Most recent AI breakthroughs have been enabled by neural networks - computing systems modelled after the human brain's neuron network. These models contain layers of simple processing units called neurons that transmit signals between layers. By tuning the neurons and connections, neural networks can identify patterns and relationships within very large datasets.
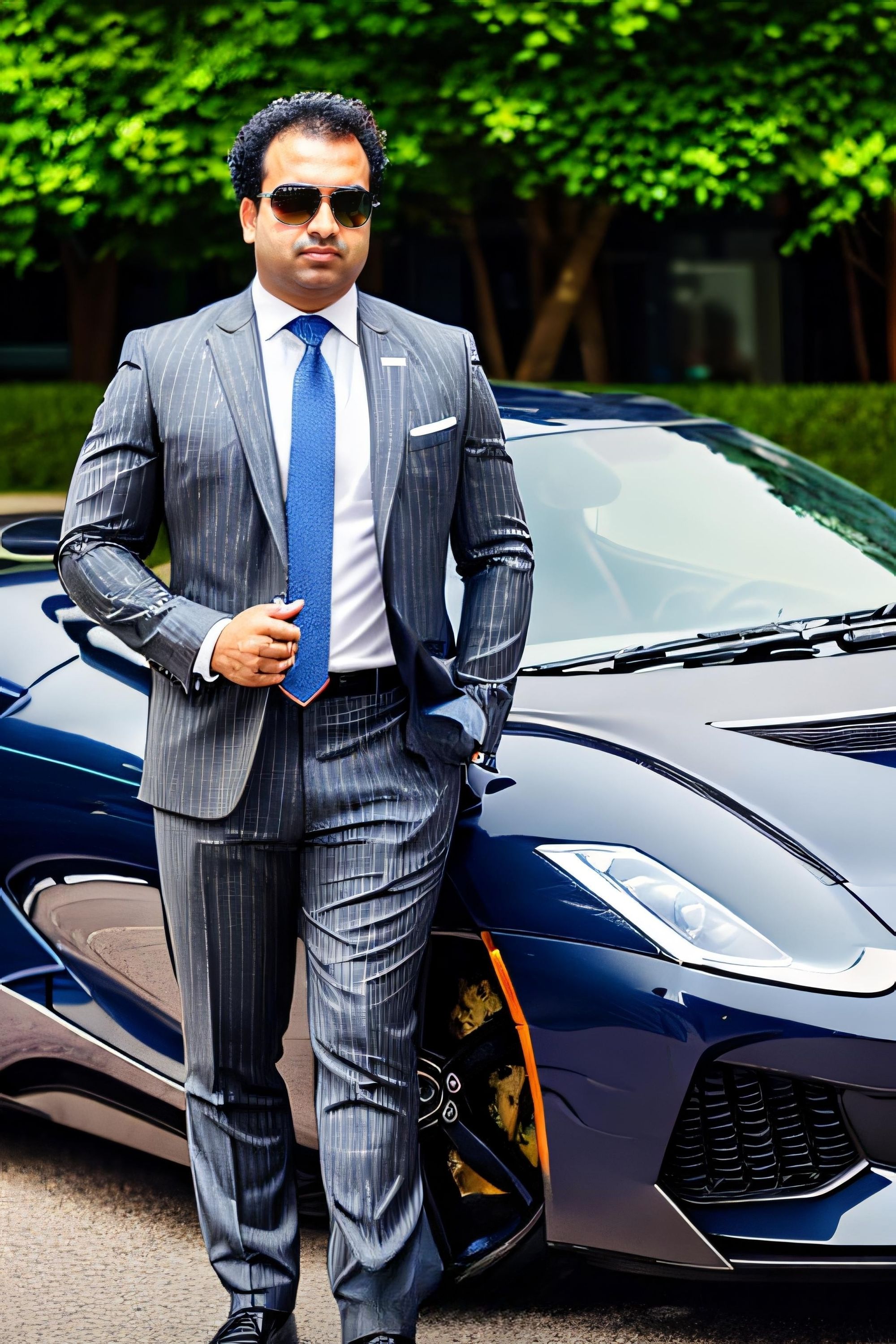
Deep learning refers to neural networks with many layers ("deep" architecture) between input and output. By chaining many simple connections, very complex functions can be modelled. For instance, an image recognition network may contain dozens of layers, each detecting different visual features - edges, shapes, and object parts. Deep learning has driven rapid advances in computer vision, speech recognition, and natural language processing.
Common Machine Learning Algorithms
Some frequently used machine learning algorithms include:
- Linear regression - Predicts continuous numerical outcomes like sales, stock prices or hospital stay length. Widely used for its interpretability and efficient computation.
- Logistic regression - Estimates discrete categorical outcomes like disease/no disease, churn or not. Useful for binary classification.
- Decision trees - Predicts outcomes by following decisions in a tree structure to their consequences. Allows modelling of nonlinear relationships and interactions.
- Random forest - An ensemble method combining predictions from many decision trees to improve accuracy and avoid overfitting.
- Support vector machines - Classifies data points by plotting them in space and identifying the gaps between categories. Performs well with high-dimensional data.
- K-nearest neighbours - Categorizes new data based on proximity to labelled training data. An intuitive, flexible approach.
- Neural networks - Can learn highly complex functions through layers of neuron connections. Includes deep learning and convolutional neural networks for computer vision.
This is just a small sample. Data scientists test multiple algorithms to determine the optimal machine-learning model for each application. More complex models become feasible as computing power expands.
The Evolution of Machine Learning
Earlier machine learning relied heavily on feature engineering - manual identification and extraction of the most relevant features from raw data by human experts. For instance, an image recognition model may use edges, corners, shapes and colours hand-selected by engineers as image features.
However, deep learning and neural networks have greatly reduced the need for feature engineering. Deep learning models can interpret raw data like images, text and audio to automatically identify patterns and abstract features needed for a task. This ability to automate feature learning has greatly expanded machine learning capabilities and applications.
In addition, deep learning utilizes much larger training datasets, enabling the identification of fine-grained patterns and nuances. Training sets have grown from hundreds or thousands of examples to tens of millions, fueled by the big data explosion. This has enabled machine learning models to interpret human speech as fluently as people and recognize virtually any object in images - impossible just a decade ago.
The Future of AI
While artificial general intelligence remains distant, focused AI capabilities are rapidly becoming powerful enough to assist people in transformative ways. Machine learning adoption has more than doubled in just the past few years as the possibilities and business value become clear across functions like marketing, healthcare, finance and transportation.
However, some argue that today's statistical machine learning will hit a ceiling. Fundamental AI progress may require exploring neuroscience-based cognitive architectures and quantum computing. Current research involves using AI itself to design better AI algorithms - a field known as "AutoML."
Whatever shape future innovations take, AI will continue advancing toward broader utility and science fiction’s visions of truly smart machines. In the near term, AI stands ready to enhance human abilities across every industry and increase our collective intelligence. Marketing is poised to become one of the foremost beneficiaries of these rapidly evolving AI capabilities.
Conclusion
This chapter reviewed artificial intelligence and machine learning concepts, history, foundations, and future directions. AI includes technologies exhibiting intelligence characteristics like learning, language processing, problem-solving and decision-making. Machine learning provides algorithms that can learn patterns from data to make predictions and decisions without programming.
Modern AI has been enabled by advances in computing power, big data, neural networks and deep learning. AI's history includes cycles of great promise and subsequent disappointment. However, machine learning now appears ready to drive real-world value across industries.
Marketers must develop a solid grasp of these foundational technologies to recognize the immense opportunities for utilizing AI in achieving business objectives. The following chapters will explore specific AI applications in marketing and illustrate how AI promises to reshape practices, workflow automation, consumer insights, personalization, engagement, and value. This ongoing AI revolution presents an exciting future for both marketers and consumers.
Chapter 2 - Key AI Technologies for Marketing
In Chapter 1, we reviewed the landscape of artificial intelligence and machine learning, including historical context and technical foundations. With that groundwork established, we now explore specific AI technologies powering the marketing transformation underway.
This chapter provides an overview of several critical capabilities and approaches within the AI domain that enable next-generation applications:
- Machine learning
- Natural language processing
- Computer vision
- Speech recognition
- Chatbots and virtual agents
For each technology, we explain what it is, review representative examples of how marketers are applying it, and assess current capabilities and limitations. Grasping these core building blocks will prepare readers for the AI marketing trends covered throughout the rest of the eBook.
Machine Learning
As discussed in Chapter 1, machine learning refers to algorithms with the ability to learn from data without explicit programming. Machine learning examines large datasets to detect patterns and make predictions or decisions. The models iteratively improve themselves by exposure to more data over time.
Key marketing applications of machine learning include:
- Predictive analytics - Forecasting metrics like sales, churn risk, lead conversion, and content engagement based on analysis of historical data.
- Personalization - Customizing content, offers, and recommendations for each customer based on preferences and behaviours.
- Anomaly detection - Identifying unusual patterns like fraud or equipment failures that diverge from normal activity.
- Sentiment analysis - Detecting subjective emotions and opinions within text data like social media, reviews and surveys.
- Image and speech recognition - Automatically identifying and processing visual and audio data.
Machine learning delivers huge gains in efficiency and performance across these use cases. However, considerable data preparation and model training is required upfront. Algorithms also lack true reasoning capabilities and can perpetuate hidden biases in data. Maintaining interpretability and auditability is crucial.
Natural Language Processing
Natural language processing (NLP) refers to AI systems designed to analyze, understand and generate human language. Key capabilities include:
- Speech to text - Transcribing spoken audio into written text.
- Text analysis - Identifying parts of speech, extracting meanings and relationships.
- Language translation - Converting text from one language to another.
- Text summarization - Condensing long pieces of text into concise summaries.
- Sentiment analysis - Detecting subjective emotions and opinions within text data.
- Natural language generation - Producing original text from data (more on this later).
Marketing applications span intelligent chatbots, analyzing customer feedback, optimizing web content, improving ad targeting through language insights, and more. However, NLP still struggles with subtleties like sarcasm and context-dependent interpretation.
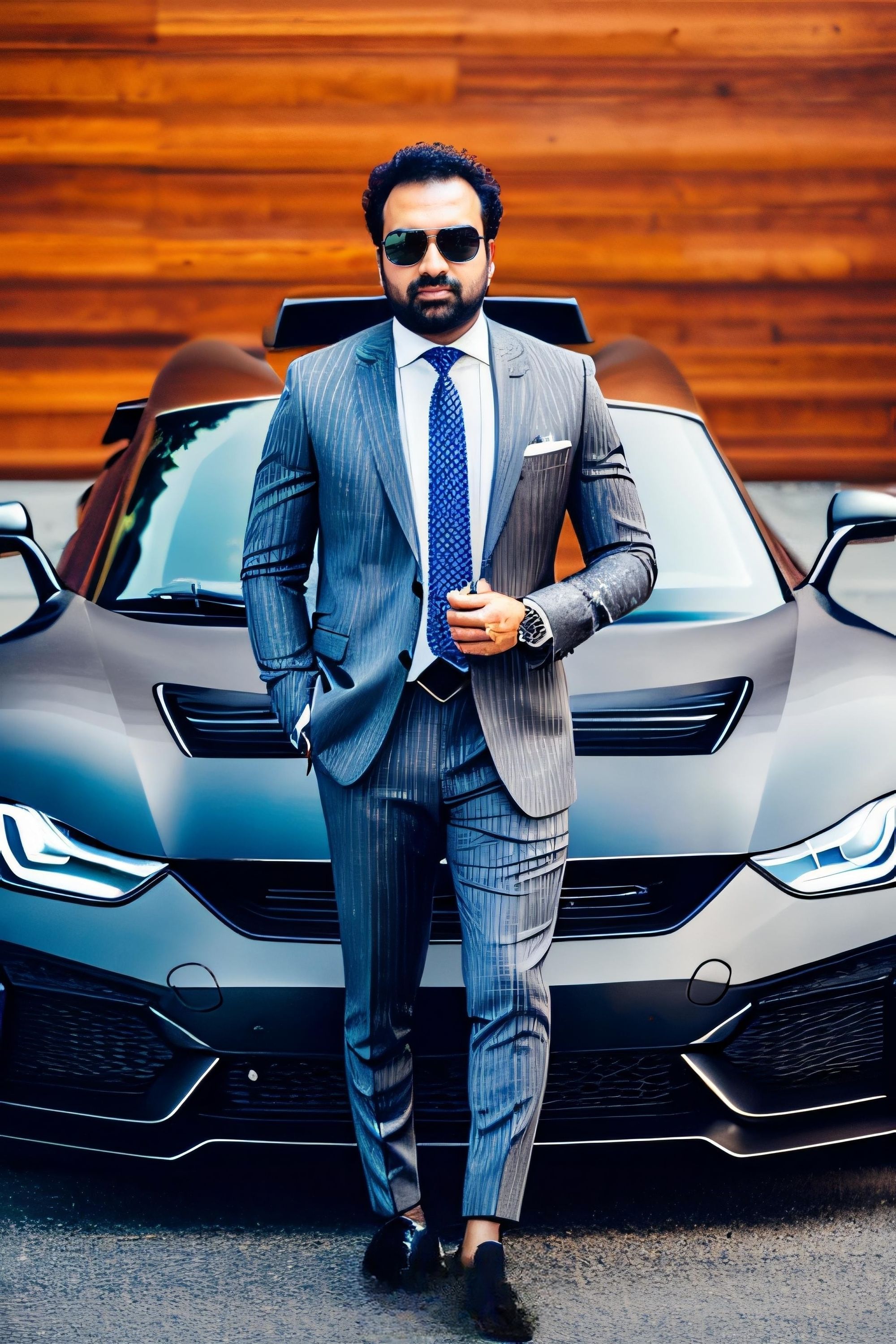
Computer Vision
Computer vision focuses on enabling AI systems to identify and understand visual content like images and video. Key capabilities include:
- Image recognition - Identifying, categorizing and labelling objects within images.
- Object detection - Locating instances of objects such as faces within images or video frames.
- Image generation - Creating original images and altering existing images.
Marketers apply computer vision in areas like ad campaign visual asset analysis, product image searches, video highlight detection, and dynamic creative optimization. However, performance varies greatly across use cases. Interpreting complex scenes and abstract concepts remains difficult.
Speech Recognition
Speech recognition aims to transcribe and understand spoken language. Key capabilities include:
- Speech-to-text - Transcribing audio recordings into machine-readable text.
- Speaker identification - Detecting and differentiating between individual speakers.
- Language identification - Discerning the language spoken from the audio input.
- Speech synthesis - Generating natural-sounding audio speech from text.
Marketing applications include voice-enabled searches, interactive voice response call analysis, sonic brand recognition, and audiobook/podcast generation. Performance has improved dramatically but still lags human abilities in noisy environments.
Chatbots and Virtual Agents
Chatbots are AI systems designed to converse with humans via text or speech interfaces. Their defining capabilities include:
- Natural language processing - Analyzing and responding to user messages contextually.
- Conversational flow - Guiding dialogues according to programmed rules and intents.
- Integration with databases and services - Accessing enterprise systems to answer questions.
- Personalization - Maintaining context on users and responding appropriately.
Chatbots are applied in marketing for customer service, lead generation, campaign management, and as brand ambassadors. However, seamless conversational experiences remain challenging. Many consumers still prefer human interactions for complex issues.
The Next Phase of AI-Powered Marketing
This whirlwind tour of core AI technologies provides the building blocks for the data-driven, hyper-personalized, and integrated customer experiences marketers envision. From predictive analytics to natural language generation, AI unlocks unprecedented capabilities. But marketers must carefully orchestrate these technologies while minding their limitations.
In the following chapters, we survey specific applications of these AI technologies powering the ongoing marketing transformation. However, AI remains a moving target, and mastering AI requires an agile, experimental mindset. Marketers must follow continuous advances across fields like deep learning and reinforcement learning to capitalize on bleeding-edge innovations.
AI implementation also demands careful data management, model governance, and ethical oversight to ensure customer trust and brand safety. AI offers immense opportunities to enhance marketing performance but also risks if deployed without sufficient human stewardship. This places a premium on marketing teams acquiring applicable skills and best practices to integrate AI responsibly and effectively.
The marketing function's full embrace of artificial intelligence will be an iterative journey, not an overnight switch. But resisting adoption risks competitive displacement. AI now clearly represents an indispensable pathway for advancing marketing excellence and propelling business success - provided its powers are harnessed judiciously.
Conclusion
This chapter explored key technologies within artificial intelligence that provide the foundation for AI marketing applications:
- Machine learning algorithms automatically improve at tasks through data experience.
- Natural language processing enables machines to analyze and generate human language.
- Computer vision focuses on automatically extracting meaning from visual inputs.
- Speech recognition converts speech to text and facilitates speech interactions.
- Chatbots provide conversational interfaces powered by the above technologies.
These capabilities unlock game-changing marketing use cases ranging from predictive analytics to personalized engagement to dynamic creative optimization. However, marketers must maintain realistic expectations regarding limitations and supplement AI with human direction.
In the next chapter, we detail how marketers are applying these technologies to transform marketing analytics using unprecedented volumes of data to extract more impactful consumer and competitive insights. Subsequent chapters explore innovative applications across content, SEO, lead management, emerging experiences like augmented reality and voice, and more. But every cutting-edge use case relies on foundational AI building blocks like those detailed here. This chapter provided the technological context to fully grasp AI's marketing potential.
Chapter 3 - AI Transforms Marketing Analytics
Artificial intelligence is empowering marketers to derive exponentially greater value from data - delivering unprecedented consumer insights to inform strategy and tactics. Advanced analytics powered by AI are enabling real-time campaign optimization, hyper-personalization, predictive modelling, and other capabilities that were previously impossible. However, with great power comes great responsibility - AI also raises pressing ethical questions around transparency and data privacy that marketers must handle appropriately as they adopt these transformative technologies.
This chapter explores the explosion of AI-driven marketing analytics and how the savvy application of analytics unlocks game-changing competitive advantage. We survey key techniques, use cases, opportunities and limitations. Properly leveraging AI analytics while safeguarding consumer trust will separate the marketing leaders of the future from the laggards clinging to outdated methods.
Consumer Behavior Prediction
Marketing analytics historically relied on segmenting consumers based on past behaviours and attributes using rules-based models. But artificial intelligence now enables truly individualized prediction of what each customer will do next - optimized in real-time.
Machine learning algorithms ingest the firehose of behavioural, transactional and contextual data from sources like web analytics, CRM systems, email, social platforms, and more. Advanced algorithms detect subtle patterns within massive datasets that are invisible to human analysts. Marketers can leverage these AI-powered insights to micro-target and personalize engagement across channels to boost response, conversion and loyalty.
For example, the same product recommendation algorithm will surface entirely different products for Customer A vs. Customer B based on their unique interests and preferences as revealed by their respective histories. AI turns the mirror from broad segments to the individual.
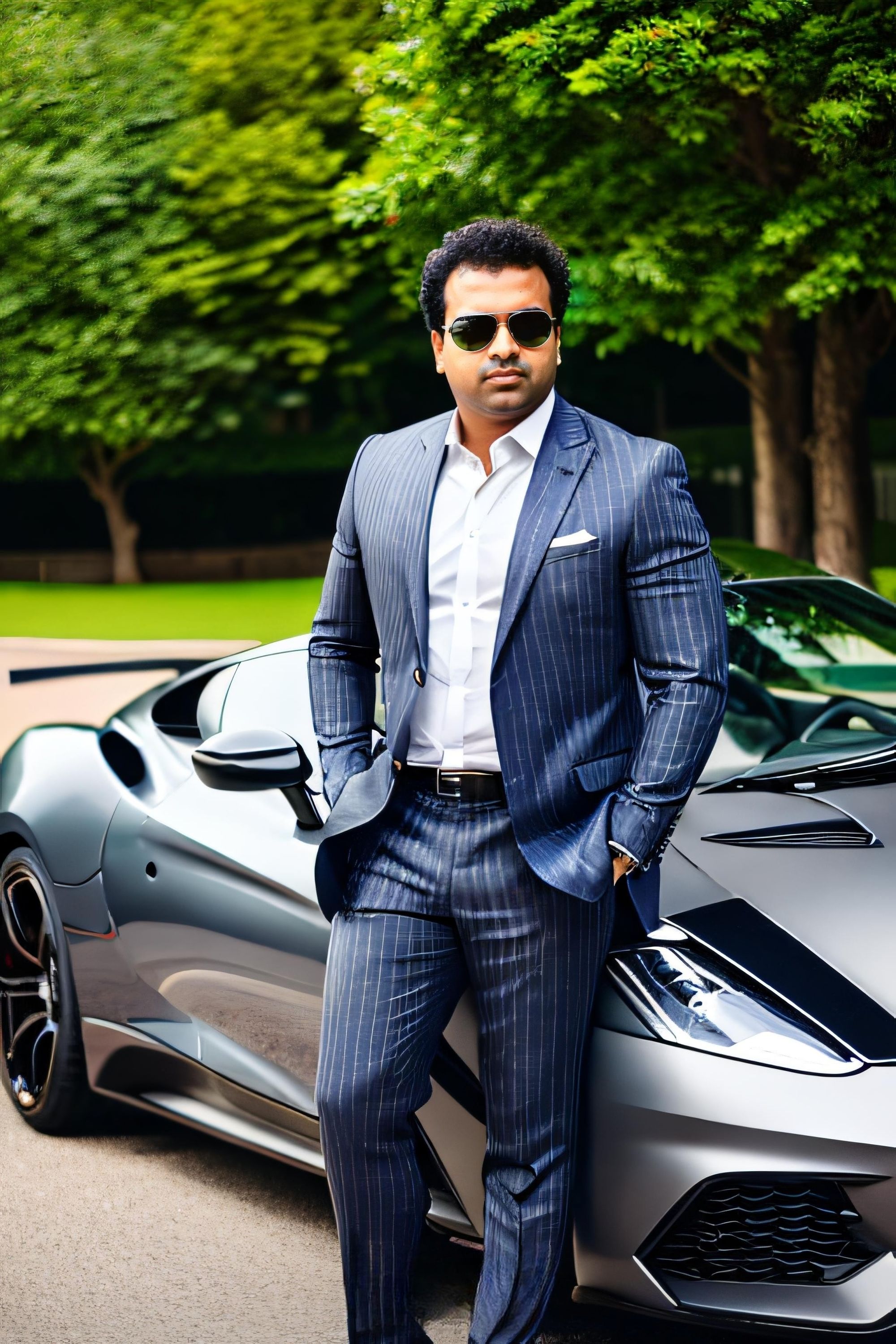
Propensity Modeling
A major application of predictive analytics is propensity modelling - estimating the probability of future outcomes like an individual's likelihood to purchase, unsubscribe, or defect to a competitor. Knowing a customer's propensities enables proactive targeting to either avoid or encourage specific behaviours.
Machine learning classifiers categorize customers or prospects based on similarity to others who exhibited particular outcomes historically. Uplift modelling is a predictive analytics technique that isolates the incremental impact specific messages or offers will have on engagement or conversion probability for a given individual. This prevents wasted marketing effort on those unlikely to be swayed.
Marketers apply propensity modelling across domains like:
- Churn prediction - Flag subscribers in danger of cancelling for targeted retention campaigns.
- Buyer propensity - Dynamically adjust e-commerce experiences for prospects closer or further from a purchase.
- Lead scoring - Route high converter prospects to sales faster and focus efforts there.
- Cross-sell & upsell - Prioritize the most relevant opportunities with each customer.
- Payment risk - Reduce fraud by screening for patterns associated with default history.
The ability to accurately predict - and directly influence - future behaviour at scale provides a critical competitive lever to marketers. But care must be taken to avoid alienating consumers with overly assertive nudging or targeting deemed "creepy." Transparency and providing clear opt-outs help maintain trust.
Sentiment & Emotion AI
Human language is nuanced, subjective, steeped in cultural contexts, and laden with sarcasm, slang, and wordplay. This makes automated analysis of unstructured text data like social media posts, reviews, and customer feedback profoundly challenging. But here too, artificial intelligence is advancing natural language processing capabilities to surface insights hidden within the subtleties of human expression.
Sentiment analysis aims to automatically detect the prevailing emotional tone or opinion within the text - whether positive, negative or neutral. AI-powered sentiment analysis provides high-level insight into brand perception and response to campaigns, products, and events across platforms. Marketers can track sentiment related to their brand and competitors over time.
Emotion AI takes text analysis a level deeper to identify specific emotions like joy, sadness, anger, disgust or surprise. This granularity provides a precise understanding of how customers feel and why. Beyond text, computer vision can now also analyze facial expressions and body language in images and video for emotional cues.
However, accurately interpreting such subjective content at scale remains an ongoing AI challenge. Nuanced human review is still essential, but AI offers a helpful radar across massive datasets. As algorithms improve, marketers gain richer awareness of the real psychological and social drivers behind consumer behaviour.
Competitive Intelligence
Marketing does not happen in a vacuum - effective strategy requires understanding the competitive landscape. Here too, artificial intelligence grants new abilities to research competitors and industry trends to inform decision-making.
AI augments competitive intelligence via:
- Social listening - Broadly monitoring competitor social media, reviews, and forums for trends.
- Web scraping - Programmatically extracting data from competitor websites.
- Ad analysis - Reverse engineering competitor ad creatives, keywords and placement.
- Pricing algorithms - Continuously optimizing pricing against market conditions and rivals.
- Predictive modelling - Anticipating competitor reactions to potential marketing plays.
AI changes competitive intelligence from periodic reporting into real-time, automated insights. The raw computing power of AI opens up new sources and depths of competitor data that were previously inaccessible. However, care should be taken to gather market data ethically and legally.
Optimizing Campaigns in Real-Time
Perhaps the most revolutionary impact of AI on marketing is enabling continuous optimization of campaigns while they are live based on real-time data. Human analysts previously had to wait until after a campaign finished before assessing performance and making tweaks for the next iteration. AI algorithms can now interpret performance data as it streams in to instantly improve campaigns in progress.
Key real-time optimization capabilities powered by machine learning include:
- Dynamic ad creative - Rotating and optimizing elements like images, copy, colours, and calls-to-action based on response.
- Media buying - Shifting ad budgets across platforms, adjusting bids and targets based on conversions.
- Web content - Changing on-page elements like headlines, layouts, and images to boost engagement.
- Search/recommendations - Updating search results or recommendations in real-time based on user data.
- Personalization - Customizing the experience for each user individually - ad creative, content, offers, pricing.
- Chat routing - Identifying intent to instantly connect website visitors to the optimal human agent.
Automating such optimizations frees overburdened marketing teams. The speed, scale, and precision of AI-driven real-time optimization create a competitive advantage.
The Power and Peril of Marketing AI
This whirlwind tour of analytical applications reflects AI's immense potential to extract transformative insights from data at unprecedented speed and granularity. However, AI also poses risks if applied without diligence and care.
On the positive side, reducing repetitive reporting and optimization tasks frees marketers to focus on strategy, creativity and building consumer connections. Data democratization makes insights accessible to inform decisions across the organization. AI also minimizes human biases that can skew judgment.
But improperly deployed analytics risk breaching consumer trust in damaging ways. Sharing or exposing sensitive data, making offensive assumptions about individuals, and aggressively manipulating behaviour rather than informing choice all represent ethical cliffs. Transparency, opt-in approvals, independent audits, and preserving human oversight help maintain integrity.
The Importance of Internal Data Literacy
A final key imperative is instilling every marketer with baseline data literacy - the ability to correctly interpret and apply analytics output. Since machine learning models do not explain their inner workings, marketers must take an active role in validating data science to ensure strategic alignment. A culture promoting transparency, accountability, and thoughtful questioning of model assumptions prevents blind faith in mathematical oracles.
With care, vision, and expertise, marketing leaders will unleash AI analytics as their secret weapon. But banishing the human element risks unintended consequences. Analytics is ultimately about serving people - a truth the brightest AI has yet to grasp.
Conclusion
This chapter revealed how artificial intelligence is augmenting marketing analytics to unlock unprecedented predictive insights, optimize campaigns, track competitive forces, and inform strategy with greater agility and precision than ever feasible before. Machine learning reveals hidden patterns within consumer behaviour and market dynamics that human analysis alone cannot perceive. AI enables real-time optimization at an enormous scale.
However, marketers must be judicious in applying these tools to avoid consumer mistrust or over-automation. Ethical usage, transparency, and human oversight help maintain integrity. With proper diligence and vision, AI analytics offer a foundation for the next stage of marketing excellence. The data to drive superior customer experiences and business results is now abundantly available. Artificial intelligence provides the key to unlocking its value.
Chapter 4 - AI Transforms Marketing Content & Delivery
Thus far, we have explored foundational AI technologies and analytical applications. Now we turn to how artificial intelligence is reinventing content creation, personalization and delivery to consumers. AI enables hyper-relevant 1:1 messages and experiences at scale - the holy grail of effective marketing.
This chapter overviews key innovations in using AI to produce tailored content, converse via chatbots, and dynamically optimize creative assets. When thoughtfully applied, AI content unlocks immense gains in customer engagement and delight. However, marketers must provide diligent human oversight to ensure brand alignment and avoid unintended offences. We survey techniques, use cases and considerations around responsibly embracing AI-powered content.
AI-Generated Text & Content
One of the most promising applications of artificial intelligence in marketing is automatic text generation using natural language processing (NLP). Given a few prompts and parameters, AI algorithms can now produce entire articles, social media posts, web content, emails, ads and other materials without human writing.
For instance, a marketer simply describes the goal, target audience and desired length. The natural language generation AI then churns out tailored content matching the intent. More advanced systems allow intricate fine-tuning of tone, style and keywords. AI copywriting platforms including startups like Jasper and established players like Google, Amazon and Microsoft already offer such capabilities.
The AI-generated text holds enormous appeal:
- Personalized content - Individualized emails, and landing pages for each lead and customer.
- Mass customization - Produce millions of unique descriptions for product inventory.
- Instant articles - Create blog posts or social media content on demand around trending topics.
- Lower costs - Reduce reliance on expensive human copywriters and content creators.
- Higher output - Automated systems generate more content at faster speeds.
However, automatically produced content poses risks including:
- Impersonal tone - AI-generated text often lacks natural personality and emotional connection.
- Formulaic quality - Outputs can feel generically templated without nuance.
- Off-brand inconsistencies - Misalignment with carefully crafted brand voice and style.
- Limited context - AI struggles to tailor language, humour and cultural references.
- Ethical issues - Potential for generated text to perpetuate harmful biases or stereotypes.
The solution is not to shun AI content due to limitations but rather apply it judiciously where appropriate and maintain human creative direction over machine outputs. AI should enhance human writers, not replace them.
Hyper-Personalized Experiences
E-commerce sites and apps are racing to provide hyper-personalized experiences using AI, tailoring every aspect to individual customer interests and context. Personalized subject lines boost email open rates. Recommendation engines suggest on-point products. Chatbots deliver customized assistance. Page layouts, content, images, offers, and pricing all get tailored in real-time.
Artificial intelligence makes such 1:1 personalization at scale achievable:
- User recognition - Identify returning visitors and recall their profiles.
- Predictive analytics - Determine customer propensities and intents.
- Dynamic content optimization - Match page content to the individual in real-time.
- Recommendation algorithms - Suggest relevant products for each shopper.
- Programmatic ad personalization - Serve tailored creative to specific personas.
- Conversational chatbots - Maintain context during multistep dialogues.
When done well, personalization dramatically boosts conversion rates and customer loyalty. However, excessive personalization risks being intrusive if pushed into unwanted tracking or manipulation. Allowing users transparency and control helps maintain trust.
Optimizing Ad Creative
Consumer attitude toward digital ads verges on outright contempt due to excessive repetition and irrelevance of most creatives. Here too, AI provides solutions via a capability called dynamic creative optimization (DCO).
DCO leverages machine learning algorithms to generate and continuously fine-tune thousands of ad variations in real time based on performance data. Elements like images, headlines, layouts, colours, and calls-to-action get remixed to identify the optimal combination that drives engagement.
Advertisers define creative parameters and let AI iterate. DCO platforms run A/B tests pitting creatives against each other, automatically cull poorer performers, refine elements that work, and launch new challengers. This evolutionary process rapidly converges on high-performance ads.
DCO represents a revolution in ad production. Madison Avenue's "Mad Men" get automated by machine learning "Math Men." In addition to heavy optimization, dynamic ads feel fresher to consumers by providing variety. Machine creativity augments human imagination.
But certain risks and limitations exist:
- Excessive novelty - Some verticals prefer stability and familiarity over dynamic creativity.
- Brand identity - Automated creative iterations may lose cohesive brand presentation.
- Ethical concerns - Unexpected AI-generated content could misrepresent products.
- Ad fatigue - Consumers may still tire of hyper-optimized creativity lacking authenticity.
For many marketing applications though, DCO delivers impressive results and its usage will only grow. But human stewardship of the process remains essential.
Chatbots & Conversational AI
One of the clearest embodiments of AI's progress is chatbots - conversational agents that interact via text or voice. Today's chatbots capably handle customer service queries, e-commerce transactions, lead qualification and a growing range of tasks once reserved for human agents.
Sophisticated natural language processing behind chatbots understands nuanced customer questions and responds contextually. Advanced dialogue systems maintain conversational context across multi-step engagements. Integration with back-end databases and applications enable accessing account information to efficiently resolve issues. Sentiment analysis allows the detection of frustration and rerouting to human agents when needed.
Chatbots offer transformative potential for better, faster, 24/7 customer engagement. However, consumers can often detect the impersonal nature of bots. Human integration is key:
- Ensure seamless hand-off to live agents when needed.
- Monitor chatbot KPIs - response time, resolution rate, and user sentiment.
- Continuously improve language processing with real user data.
- Program charming personality without excessive anthropomorphism.
- Write tight dialogue rules aligned with brand voice versus generically templated responses.
When thoughtfully implemented, chatbots enable brands to cost-effectively scale exceptional engagement.
The Content Imperative for AI Marketing
Content remains essential - AI should enhance human creativity on this front, not aim to fully supersede it. The unique value of content produced by and for people remains irreplaceable. AI's role is multiplying human content capabilities, maximizing relevance, iterating message variants, and freeing teams for higher-order tasks.
But relinquishing all human oversight risks brand reputation and consumer trust. While AI content holds enormous potential, keeping 'heart' at the centre is critical. At the end of the day, marketing is about meaningfully connecting with people. Artificial intelligence that loses sight of the humanity it serves to amplify risks detached, robotic outcomes.
Conclusion
This chapter revealed how AI is transforming marketing content creation, personalization and delivery:
- Natural language generation automatically produces text tailored to goals.
- Hyper-personalized experiences boost engagement and conversion.
- Dynamic creative optimization iterates high-performing ads.
- Chatbots enable conversational interactions.
Thoughtfully integrating such capabilities multiplies human creativity. But retaining human oversight ensures branding consistency and avoids consumer alienation. Core human connection should remain the compass guiding AI innovation.
The next chapter explores AI applications in critical marketing capabilities like SEM/SEO, email marketing and campaign management. AI reveals insights that optimize programs for superior performance. But strategy should direct technology, not vice versa. Fulfilling marketing's true purpose requires a balance between automated intelligence and the human heart.
Chapter 5 - AI Optimizes Core Marketing Capabilities
We have now surveyed several primary domains where artificial intelligence is transforming marketing - from analytics to content creation. This chapter explores how AI is optimizing core marketing capabilities including search, email, and cross-channel campaign management. AI reveals insights and automates tasks to boost productivity and performance across these essential programs.
We will examine the growing role of AI in areas like:
- Search engine optimization
- Pay-per-click & digital ads
- Email marketing
- Marketing automation
- Campaign management
Implemented judiciously, AI can streamline these workhorses of the marketing stack to operate with enhanced precision, personalization and automation. However, marketers should maintain realistic expectations regarding AI’s limitations in augmenting, not fully replacing, human creativity and oversight.
AI for Search Engine Optimization
Search engine optimization (SEO) is a chief responsibility for most marketers seeking visibility for their brand, products and content. AI is taking on an increasing role optimizing websites for higher rankings by analyzing page content, links, metadata, user engagement signals and more.
Specific AI techniques improving SEO include:
- Natural language processing to optimize page content and meta descriptions for chosen keywords.
- Sentiment analysis to assess and enhance emotional resonation of copy.
- Automatic meta tag population and site linking to boost crawlability.
- Image and video analysis to add descriptive alt text and captions.
- Tracking rankings in real-time and correlating factors driving fluctuations.
- Predictive modelling to estimate traffic lift from targeting new keywords.
- Analytics to pinpoint on-page elements that boost dwell time and engagement.
As with all marketing AI, oversight is required to align AI-driven optimizations with branding strategy versus blindly chasing rankings. The aim should be crafting high-quality experiences that both users and algorithms appreciate.
Pay-Per-Click & Digital Advertising
Paid digital advertising relies heavily on testing and optimization. Here too, AI lends its capabilities:
- Algorithmic bid optimization adjusts bids to maximize conversions within budget constraints.
- Ad creative optimization dynamically iterates elements in real-time to improve performance.
- Predictive modelling helps forecast the performance of new ad variants or placements.
- Anomaly detection identifies sudden changes in key metrics requiring intervention.
- Automated ad generation creates new display ad combinations matching parameters.
- Competitive insights reveal trends in competitors’ bidding behaviour and creatives.
AI reduces manual optimization workload for PPC and frees marketers to focus on strategy and creative direction. However, blind reliance on black box bid algorithms risks losing an understanding of what is driving performance. Maintaining human governance prevents over-automation.
Revolutionizing Email Marketing
Many hail email marketing as “dead” but savvy marketers know it remains a core channel. AI again brings new life with capabilities like:
- Natural language generation to create customized email content tailored to each subscriber.
- Predictive analytics to forecast subscriber engagement and target accordingly.
- Message personalization with dynamic content blocks tailored to the individual.
- Subject line and send-time optimization based on response modelling.
- Recommending email segments to remove or add users based on activity.
- Automated email triggers customized based on events and user data.
- Chat analysis to categorize subscriber questions and improve FAQs.
Applied judiciously, AI enables more relevant, timely and personalized subscriber communications. But marketers should be wary of “over-AI” resulting in generically templated emails devoid of human touch. Preserving genuine connection matters most.
Smarter Marketing Automation
Marketing automation platforms aim to streamline campaign execution and messaging. AI improves key capabilities:
- Predictive lead scoring models to identify high-potential prospects.
- Hyper-customized nurture tracks tailored to individual leads.
- AI content creation for personalized emails, landing pages, and ads at scale.
- Intelligent list segmentation using machine learning models.
- Real-time alerting of engagement changes to trigger responses.
- Event-based and behavioural audience suppression to avoid over-messaging.
Again, oversight is key as excessive automation risks irritating customers and prospects with hyper-targeted nonsense. Automation should aim to humanize marketing, not replace people.
Orchestrating Cross-Channel Campaigns
The holy grail for marketers is orchestrating integrated campaigns spanning channels, teams and technologies. Here AI delivers value:
- Central campaign data lake for unified analytics and reporting.
- Attribution modelling to quantify channel contribution to goals.
- Predictive analytics for more accurate budget and result forecasts.
- Workflow automation for executing repetitive cross-channel tasks.
- Real-time alerting when campaign metrics diverge from the plan.
- Recommended optimizations across programs based on insights.
With 360-degree customer views and automation, AI helps align disparate efforts. But only human leadership can define an integrated strategy and creative vision across fragmented systems. Technology supports but cannot replace unity of purpose.
Maximize Marketing ROI with AI
This survey of core channels reveals that when applied strategically, AI can optimize myriad marketing activities for superior performance. Democratizing insights across teams and automating tedious tasks improves productivity and outcomes.
But blind overreliance on algorithms will fail to achieve lasting competitive advantage. The essence of marketing remains profoundly and beautifully human. AI must serve that core purpose with diligence and humility.
Marketers who maintain realistic expectations of AI, and consult its counsel but follow their own vision, will maximize marketing ROI. But those who check their human judgment at the machine learning door cede irreplaceable wisdom. AI alone cannot quantify life’s most important values.
This is not a Luddite argument against technological progress but rather a reminder that marketing, done right, speaks to the soul. The path ahead requires integrating automation with humanity's highest aspirations. As marketers, we face an unprecedented opportunity to uplift customers, communities, and the world. If AI supports that ambition, virtue will manifest.
Conclusion
This chapter revealed how AI is transforming core marketing capabilities and channels including:
- Search engine optimization
- Pay-per-click & digital ads
- Email marketing
- Marketing automation
- Cross-channel campaign orchestration
AI delivers insights and automation to streamline these workhorse programs for greater productivity and performance. However, human creativity, ethics and strategy must remain the north star guiding AI optimization.
In the next chapter, we explore how AI is reshaping marketing sales processes - from lead generation to sales recommendations and customer hand-off. AI can target and nurture high-potential leads but also risks alienating consumers if applied unethically. Maintaining human oversight and wisdom ensures marketing technology supports noble purposes.
At its best, marketing has a profound impact on lives and society. As AI capabilities grow, we must uphold our humanity and highest values. Doing so will maximize marketing ROI and positive impact for the benefit of all.
Chapter 6 - AI Redefines Marketing Sales Processes
Beyond powering analytics and customer engagement, artificial intelligence is also transforming sales processes - from attracting and scoring leads to sales recommendations and hand-offs. This chapter explores the growing role of AI in boosting qualified lead generation, predicting conversion potential, recommending cross-sells and upsells, and autonomously nurturing leads until sales-ready.
When applied strategically, AI sales capabilities enable more efficient and effective lead funnel management, sales forecasting, opportunity prioritization, and sales representative performance. However, marketers must thoughtfully weigh the benefits against the risks of overly aggressive automation alienating customers. Maintaining human oversight and wisdom ensures marketing technology supports, rather than overrides, human needs.
Smarter Lead Generation
Inbound lead generation is a pillar of marketing ROI. Here, AI lends its capabilities in areas like:
- Web activity analysis - Identifying high-intent website visitors based on behaviours.
- Chatbot lead capture - Using conversational interactions to capture lead details.
- Predictive lead scoring - Modeling contact propensity to convert based on attributes.
- Automated lead enrollment - Triggered by events like site activity.
- Personalized lead nurturing - Customized journeys for each prospect.
- Sales process optimization - Analyzing successes and failures to improve funnel.
When integrated with CRM and sales systems, AI-driven lead gen provides higher-quality prospects to sales teams. However, overly aggressive automation can irritate consumers. Ensuring transparency and user control preserves trust.
Predicting Lead Conversion Potential
Not all leads are equal. AI predictive lead scoring helps focus sales efforts on the hottest prospects by analyzing attributes of past converted leads to identify patterns. Models predict conversion likelihood for new leads based on similar qualities.
Typical attributes assessed include:
- Firmographic data - industry, company size, location etc.
- Behavioral signals - email opens, page views, content downloads.
- Marketing interactions - campaign touchpoints, form fills, ads clicked.
- Customer lifetime value - potential value based on persona.
- Engagement metrics - email clickthroughs, link clicks, time on site.
Predictive lead scoring enables automatically routing higher potential leads to sales faster. However, over-reliance on models risks misjudgments or bias. Human oversight ensures lead prioritization aligns with strategic growth goals.
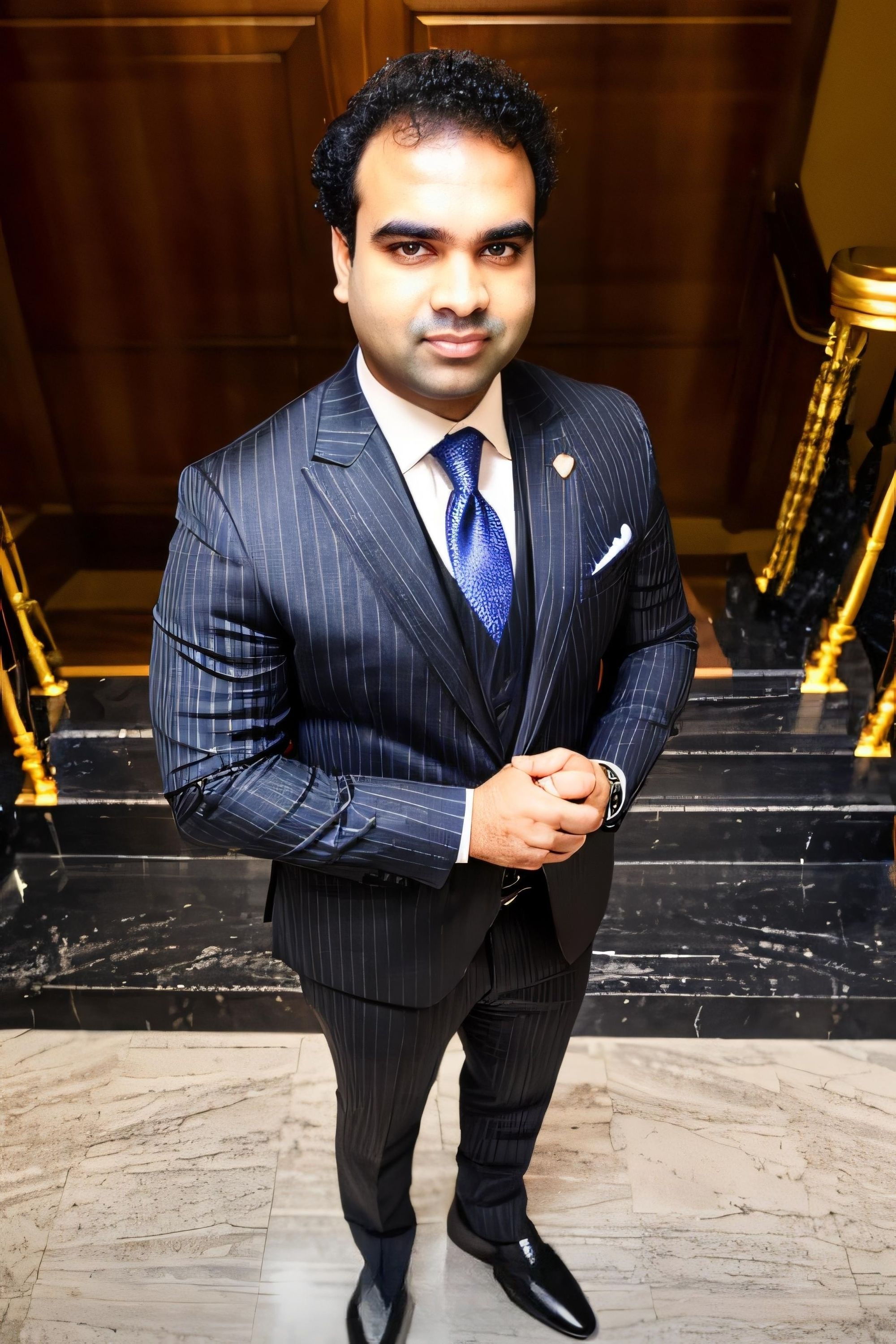
AI Sales Recommendation Engines
Beyond scoring incoming leads, AI powers sales recommendation engines that suggest relevant products, services and content drive conversions. Recommendations get personalized based on factors like:
- Past purchases
- Website activity
- Browsing history
- Stated preferences
- Demographic data
- Cross-sell models
- Churn probability
- Expected lifetime value
AI recommendations can boost order values. But excessive intrusiveness and unwanted upsell risk customer frustration. Allowing user transparency and opt-out preserves trust.
Automated Lead Nurturing
Basic marketing automation platforms already automate workflows for scoring leads and triggering nurture campaigns. AI augments these capabilities for hyper-personalization:
- AI content generation creates customized emails, landing pages for each lead.
- Predictive analytics model the optimal communications mix and cadence for each lead.
- Machine learning classifiers continuously improve lead segmentation and targeting.
- Natural language generation produces tailored content addressing prospect needs and interests.
- Virtual agents provide 24/7 customized sales support via chat.
When orchestrated strategically, AI-powered lead nurturing provides a high-touch, automated experience boosting conversions. But again, human oversight is required to ensure empathy and relevance that avoids irritation.
Facilitating Hand-Off to Sales
CRM systems aim to ensure smooth transitions from marketing qualified leads to sales accepted opportunities. AI enhances this through:
- Lead scoring helps clearly identify sales-ready prospects based on automated criteria.
- Natural language processing analyzes sales call transcripts to improve hand-off insights.
- Predictive modelling forecasts expected revenue from each lead to inform sales prioritization.
- AI virtual agents can book meetings or instantly connect highly qualified prospects to reps.
- Monitoring outcomes provide feedback loops to continuously improve the marketing to the sales funnel.
AI enables insights to facilitate smarter lead transitions to sales. But strategy must ensure technology tracks human needs and emotions, not just metrics. Marketing and sales share a noble duty - serving people. AI is a means, not the end.
The Future of AI Sales Automation
This overview reveals how AI shows immense promise for optimizing the lead-to-revenue engine and sales processes. Integrating marketing systems with sales CRMs via AI enables unified analytics, shared insights and coordinated workflows. Repetitive sales tasks get automated while reps focus on relationship building.
However, marketers must weigh the benefits against the risks of over-automation in sales contexts. If allowed to minimize human relevance, marketing AI risks unintended detrimental consequences like irritating consumers, degrading brands and undervaluing human dignity. Technology should aim to uplift people.
When applied judiciously, AI sales capabilities create a competitive advantage. But human wisdom, ethics and oversight remain imperative to ensure marketing AI supports noble purposes. At its best, marketing and sales professions represent a noble calling to serve. AI offers tools to amplify our impact but wisdom determines how we wield them and to what ends. Our future rests in that choice.
Conclusion
This chapter revealed how AI is transforming sales processes including:
- Smarter lead generation
- Predicting lead conversion
- Sales recommendation engines
- Automated lead nurturing
- Facilitating hand-off to sales
Thoughtfully integrating AI enables more optimized, personalized and predictive sales funnel management. However, human creativity, ethics and strategy must remain the guiding forces to ensure marketing technology aligns with serving human needs.
In the next chapter, we will explore emerging AI applications like personalized product recommendations, emotion detection, voice-based engagement and more. While promising, these nascent capabilities warrant prudent evaluation to determine if, when and how they should be leveraged. Marketing AI holds immense potential but also risks if decoupled from human wisdom. Our future demands we apply new technology judiciously and maintain our humanity.
Chapter 7 - Emerging AI Marketing Applications
Thus far we have explored core AI capabilities powering evolution in marketing analytics, content, sales processes and more. Now we look ahead to emerging applications that promise to further transform marketing in the coming years.
This chapter surveys a sample of nascent AI innovations including:
- Personalized product recommendations
- Emotion detection
- Augmented reality experiences
- Voice-based engagement
- Connected TV and digital audio
- Intelligent retail environments
These expanding use cases present exciting potential but also warrant prudent evaluation. Marketers must weigh benefits against risks, and challenges in implementation, to determine if and when adoption is merited. Maintaining realistic expectations of still-evolving technologies while envisioning their future possibilities will enable marketers to capitalize on AI innovation emerging over the horizon.
AI-Powered Product Recommendations
One of the most pervasive AI applications is automated recommendation engines that suggest relevant content and products to users. These algorithms analyze behavioural data, contextual cues, and product attributes to create highly personalized recommendations.
Example data inputs include:
- Purchase history
- Browsing behaviour
- Search keywords
- Product views and ratings
- Demographic data
- Inventory and availability
- Comparison with similar users
Sophisticated algorithms determine optimal product relationships and relevance for each user in real-time. The AI models continuously improve based on recommendation performance.
Product recommendations powered by AI deliver multiple benefits:
- Higher sales from suggesting on-point products
- Improved customer experience and engagement
- Showcasing full product catalogue, aiding discovery
- Higher average order value via cross-sell opportunities
However, excessive or low-relevance recommendations risk annoying users. Maintaining transparency and allowing user feedback helps algorithms improve.
Emotion AI for Marketing
Emotion detection is an emerging domain within artificial intelligence. Using computer vision, biometrics, machine learning and natural language processing, emotion AI aims to understand human emotional states and sentiments.
Marketing applications for emotion AI include:
- Facial analysis - Gauging reactions to ads, content and experiences by scanning facial expressions.
- Tone analysis - Detecting anger, joy or sarcasm in conversational interactions.
- Body language reading - Discerning engagement, confusion or disagreement from poses.
- Affective computing - Adjusting experiences dynamically based on sensed emotions.
- Sentiment analysis - Identifying themes and emotions within open-ended feedback.
Emotion AI shows promise for quantifying subjective responses to improve engagement. But substantial technical hurdles remain in accurately interpreting such nuanced human states. Ethical risks around privacy and emotional manipulation must also be managed carefully as these capabilities evolve.
Immersive Augmented Reality
Augmented reality (AR) overlays digital information and experiences onto the physical real-world environment. AR is progressing rapidly from a novelty to a sophisticated tool for brands.
Marketing applications enabled by AR include:
- Enhanced product visualization - Overlaying layers of data onto physical products.
- Immersive branded environments - Creating engaging AR worlds for customers to explore.
- Product configuration - Customizing physical goods with AR digital overlays.
- Contextual in-store offers - Launching AR coupons, animations and info triggered by location.
- Gamification - Adding AR challenges and rewards to brand interactions.
AR provides multidimensional engagement not possible in conventional media. But marketers must balance innovation with practical utility. Seamless AR adoption remains constrained by hardware limitations today. But rapid smartphone advances will likely make AR ubiquitous within years.
Optimizing for Voice Engagement
Voice-based interfaces are going mainstream through smart speakers and voice assistants like Alexa and Siri. Brands must optimize for vocal engagement as consumers increasingly use voice search instead of typing.
Key opportunities in voice marketing enabled by AI include:
- Optimizing content for voice search - Using natural language generation to improve visibility.
- Voice ads - Creating highly targeted audio ad content.
- Voice apps - Developing utility-driven brand apps on smart speaker platforms.
- Personalization - Customizing responses based on individual identity and context.
- Conversational commerce - Using natural dialogue for product discovery and purchases.
- Sentiment analysis - Detecting vocal indicators of frustration to improve voice UX.
Voice provides a natural but challenging new interface. Marketers must focus on utility over gimmicks in this channel. But refined vocal interactions powered by AI conversational ability offer a promising new engagement frontier.
AI Across Digital Media
Innovations in digital media open new avenues to engage consumers with AI-driven experiences. For example:
Connected TV - Streaming television powered by integrated internet allows interactive TV ads. AI enables:
- Automated video ad optimization for performance.
- Predicting viewer engagement and serving dynamic creative.
- Contextual ad targeting based on viewing behaviour.
Digital audio - Music and podcast platforms enable advanced audio ad experiences. AI capabilities include:
- Optimizing audio ad sequencing and personalization using voice biomarkers.
- Predicting engagement through sentiment analysis of reactions.
- Generating personalized audio ads dynamically tailored to listeners.
AI unlocks richer data and flexibility to optimize emerging digital media environments in innovative ways. But as always, marketers should focus on value, not technology for its own sake.
Intelligent Retail Environments
Brick-and-mortar retail is being reimagined through immersive AI environments fine-tuned to shopper actions and emotions.
Examples include:
- Cashierless stores powered by sensor fusion tracking purchases.
- Recommendation engines proactively offering products.
- Emotion AI gauging reactions to inform store improvements.
- Contextual promotions based on location, time and buying history.
- AR product visualizations overlaying interactive digital data.
Futuristic retail showrooms by companies like Amazon Go fully integrate physical and digital through AI. Intelligent automation enables more efficient operations while data-driven personalization boosts sales. But concerns around privacy risks remain.
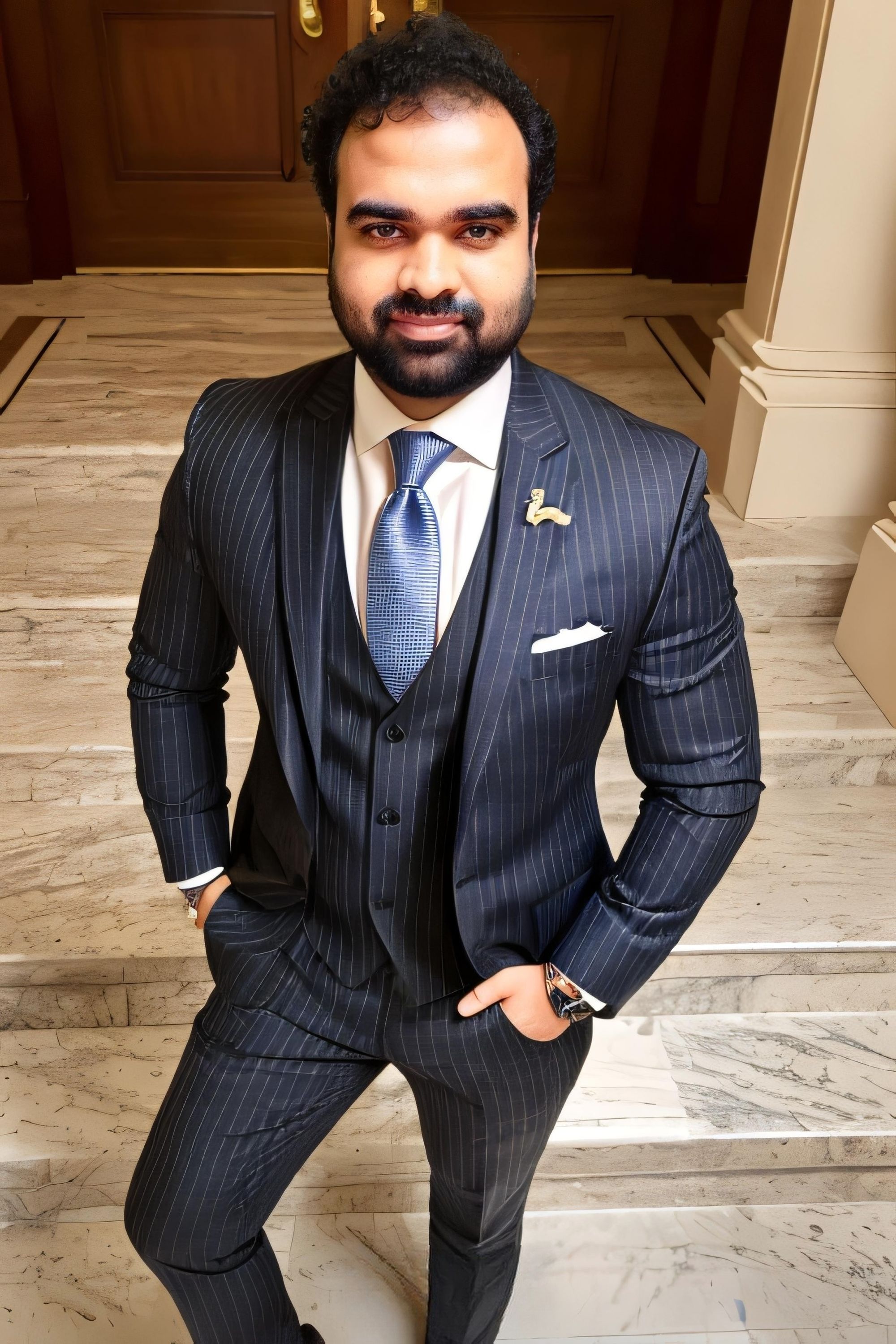
Prudent Innovation for Marketing AI
This sampling of emerging capabilities reveals AI's potential to transform marketing in the coming years. However, marketers should approach newly hyped innovations with a prudent framework evaluating if, when and how they might merit adoption given limitations today. Preserving realistic expectations helps build appropriate AI strategies.
The priorities should be enhancing genuine consumer value and relationships versus novelty or buzz. AI implementation demands careful data governance, ethics and alignment with brand objectives.
But by prudently yet boldly embracing AI innovation, forward-looking marketers can accelerate competitive advantage. The future remains unwritten - wise application of AI advances helps manifest an uplifting vision.
Conclusion
This chapter highlighted emerging AI marketing applications to envision possibilities on the horizon:
- Personalized recommendations
- Emotion detection
- Augmented reality
- Voice engagement
- Digital media experiences
- Intelligent retail environments
Thoughtful innovation combining human imagination and AI capabilities will unlock new ways to meaningfully engage customers. However, marketers should evaluate each emerging technology on its merits with realistic expectations before pursuing adoption.
In the concluding chapter, we step back to consider the broader implications of AI for marketing. Opportunities abound to elevate the profession through AI but also risks if adoption lacks strategy and wisdom. Marketing leaders must chart a course that applies AI judiciously to maximize its benefits while maintaining enduring human values. Savvy marketers who effectively leverage these technologies will gain a substantial competitive advantage. This transformation promises to be both disruptive and empowering for the field.
Chapter 8 - The Future of Marketing in an AI World
We have now surveyed the landscape of how artificial intelligence is transforming marketing - from foundational technologies to analytics, content creation, campaign management and emerging applications. In this concluding chapter, we step back to consider the broader implications of marketing’s AI revolution.
Marketing is on the cusp of a new era - one of exponentially greater productivity, consumer insights, creative possibility and human augmentation. But also one fraught with unprecedented ethical challenges, demands for new skills, and risks of over-automation. How marketers navigate this transition will determine whether AI elevates the profession to new heights or leads it down an unintended path.
This chapter will contemplate key questions and implications marketers must grapple with to direct AI's impact toward uplifting outcomes.
Reimagining Marketing’s Core Value
The acceleration of artificial intelligence begs reflection on the very purpose and essence of marketing. Is the function’s highest aim perfect predictive behavioural modeling and meticulous media execution? Or is it building meaningful human relationships, trust, and delivering joy?
AI empowers marketing processes unimaginable just years ago. But technology alone cannot foster emotional bonds, empathy, or creativity. As machine capabilities grow more powerful, preserving marketing’s soul becomes only more imperative.
The responsibilities most sacred to marketing’s enduring purpose must remain in human hands - discerning emotional and social insights, synthesizing the unique imaginative sparks that touch hearts and minds, and weighing ethical implications of how data and targeting affect lives. This is marketing’s spiritual core.
But many mundane tasks can be delegated to AI - data cleaning, media buying, personalization, lead scoring, message delivery, and reporting. The technology handles execution, freeing people to focus on human relationships and strategy.
A wise marketer sees this boundary. Saving the humans where they are irreplaceable preserves room for the magical essence only we can provide. Leadership means ensuring both AI efficiency and enduring human wisdom harmonize in service of social good.
New Skills for the AI-Enabled Marketer
Maximizing the partnership between human creativity and machine productivity demands marketers evolve new skills. Technical fluency will become essential - not to supplant data scientists, but rather to properly frame objectives, audit models, validate output and course-correct appropriately.
Key capabilities the modern marketer will require include:
- Data literacy - Basic fluency in statistics, analytics and metrics.
- AI fundamentals - High-level grasp of how technologies and models work.
- Ethics training - Understanding risks of bias, transparency, and privacy.
- Strategic thinking - Framing problems and desired outcomes, not just tactics.
- Creativity and empathy - Unique human gifts resistant to automation.
- Change management - Leading people through transformations.
- Critical inquiry - Asking tough questions, avoiding blind faith in machines.
- Business strategy alignment - Ensuring all efforts deliver the desired value.
This blend of technology fluency and human-centred skills enables marketers to be accountable, proactive partners to data scientists and engineers while retaining focus on customer experiences.
Rethinking Day-to-Day Marketing Work
The tactical activities occupying marketing teams will shift substantially in an AI-enabled paradigm. Consider core marketing workflows:
Campaign management transitions from manually pushing buttons to overseeing automated, predictive orchestration powered by machine learning optimization. Rather than doing the tasks, marketers curate dynamic systems.
Content creation moves from solitary human authoring to collaboratively guiding AI algorithms generating customized narratives - a partnership between human creativity and machine productivity.
Consumer insights get revealed instantly through automated analytics encompassing massive behavioural datasets, not periodic reporting summarizing survey samples. Continuous feedback optimizes strategy.
Media planning goes from demographic segmentation and static allocation to modelling response propensities for each individual in real time across infinite channels. Campaigns continuously adapt.
Even emotional copywriting may begin not from a blank page, but rather prompts to an AI system trained on brand voice and objectives. The machine brainstorms creative concepts powered by human direction.
Thinking more broadly, consider how strategy evolves from periodic brainstorms to an agile, iterative process fueled by endless AI-generated hypotheses to test. Intuitive judgment gets augmented by trusted machine counsel.
Truly realizing such exponential gains demands a workforce mindset shift. People’s highest value skills must direct technology while letting algorithms handle repetitive tasks. This frees the humans within marketing to pursue more creative, collaborative, and strategic peak human potential combines with liberated machine productivity.
But friction emerges if specialists cling to old ways. Adaptability, willingness to automate the automatable, and trust in AI colleagues pave the smoothest path ahead.
Pressing Ethical Challenges
Despite AI’s enormous potential for progress, its application poses ethical risks requiring diligent mitigation. Marketers must grapple with complex issues including:
- Data Privacy - Collecting and securing immense consumer data stores necessitates security, transparency and providing choice where possible.
- Algorithm Bias - Models can inherit and amplify prejudices buried within training data. Diversity and oversight help.
- Transparency - Explainability, auditability and accountability should be baked into black box AI systems.
- Personalization Ethics - Relevant personalization must avoid intrusive “surveillance marketing” violating consent.
- Business Disruption - AI could render many functions and jobs redundant. Transition support helps mitigate fallout.
- Tech Addiction - Over-reliance on machines risks eroding human wisdom and judgement.
- Manipulation - AI could coerce destructive or excessive behaviours absent oversight.
- Misuse - Criminals and repressive regimes might exploit consumer profiling or media control.
There are no perfect solutions, but conscientious leaders can help steer AI toward positive progress by establishing ethical guidelines, governance, employee education, and championing transparency. Keeping people at the centre is critical.
An Opportunity to Uplift the World
Applied judiciously as a tool aligned with noble goals, artificial intelligence can uplift marketing as a force for good like never before. But unseriously applied, it risks unintended regression. This is no exaggeration - technology of such immense powers demands perspective.
Some possible positive visions of an AI-accelerated marketing future:
- Next-generation customer experiences packed with value
- Democratizing services through increased accessibility
- Hyper-relevant and always-on education empowering consumers
- Fostering deeper human relationships and community
- Platforms giving voice to diverse populations
- AI augmenting human creativity and passion
- Leaps in business innovation expanding prosperity
- Marketing aligned with social responsibility
Realizing this potential requires mindset shifts. The technological possibility must bow to human wisdom. Business objectives matter but only alongside social impact. And creativity should direct algorithms, not vice versa.
An inspired future beckons but remains in our hands to manifest. The only limits are those of imagination and will.
Conclusion and Final Thoughts
And so our AI journey comes full circle. We have surveyed marketing’s technology revolution from foundation to vision. How swiftly the future comes into focus.
Artificial intelligence promises to amplify marketing beyond our imaginations - perfecting analytical precision, unlocking creativity, and maximizing consumer value. Applied judiciously, AI can elevate marketing to unprecedented positive influence.
But carelessness invites unintended consequences. Absent human oversight, even the most powerful machine devolves into darkness.
What matters most now is setting AI on the right course. Leaders must champion ethics, vision, and wisdom - called ever more to that sacred duty as progress accelerates.
Marketers play a central role in determining whether AI uplifts humanity or diminishes our spirit. But living future history through this transformation, we must believe in the light.
Flanked by a reflective pause and bold imagination, marketing's path ahead appears bright. Though questions remain many, answers emerge in time. Faith steels our nerves.
And so with cautious optimism we embark, heart engines stoked, conviction high, minds sturdy. The future awaits our steerage. AI but the wind beneath marketing's soaring wings.
Onward.